10 Pieces of Advice on Data & AI I Hate
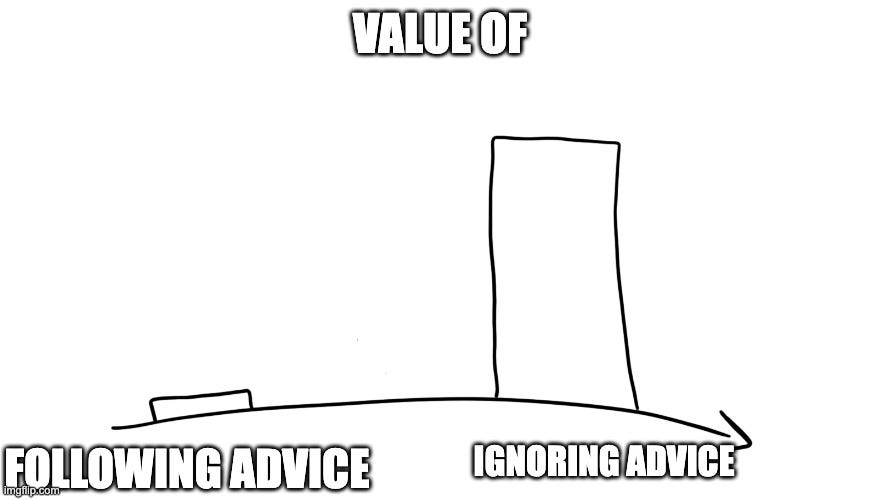
If you hear any of the following ten statements, I want you to stop and think.
Here goes my top ten list of statements that don’t apply to most companies and people:
Everyone has to get into data & AI.
That’s true, broadly speaking.
But it is just as true as “every business should have a good business strategy.”
But now what? Now nothing.
I hate this piece of advice because it’s not actionable at all!
I think much better advice is to start examining how you could incorporate data and AI into your core business strategy.
If you can’t, you should probably not start with data and AI right now but instead work on your business strategy.
Start with data engineering and business intelligence; AI & ML will follow.
I don’t like this advice because there’s no evidence it works.
Yes, in the past, when no one was doing machine learning, companies adopted business intelligence and data engineering.
Machine learning followed because it became feasible from a technological standpoint.
That doesn’t mean that companies today have to endure the same troubles they did a decade ago.
Today, I think the best advice is to start with whatever best fits your business, which may very well be a machine learning-based application rather than business intelligence.
Hire the right people, and they will take care of it.
You need to hire the right CDO, Head of Data, or CIO, and he’ll ensure your data & AI efforts are rolling, right?
Except they won’t.
Hiring great software engineers and a good CTO is a must for building great products, but it’s the product managers and the business people who shape the actual product strategy.
It’s the same for data & AI. You need product managers with great insights into data & AI to make progress in that field, too.
If you truly want to move into data & AI, you’ll need to hire a good cross-functional team, including business-minded individuals, whether they call themselves “leaders” or “PMs.”
Start with your own data.
It’s a good argument: You already have tons of proprietary data, so you should build your AI product on top of that.
Most great data products are built on data companies' own, like Airbnb search algorithms or Netflix's recommendation system.
But this argument overlooks an important fact: Most companies also invest heavily in data collection, either by gathering it themselves or buying it.
Of course, then they have it, but when they start building their data product, they might not.
You don’t need to start with your data at all. It’s an investment decision. If you need to collect data to build something twice as good, please invest!
AI, ML, and LLMs are overhyped, so you shouldn’t use them.
Yes, there’s a hype cycle, and yes, LLMs and other AI technologies are probably overhyped.
But that doesn’t mean you shouldn’t use them. It just means you have to run your own analysis, which you have to do anyhow.
The fact is, AI, ML, and LLMs have leapfrogged in front of us. There’s been huge progress in multiple fields; it’s simply stupid not to utilize this set of amazing tools.
You may have good opportunities to utilize these technologies. Please don’t ignore them; run your analysis, start your data initiatives, and build great products.
Use data to solve your existing problems.
I hear this advice all the time: to start out with machine learning, why don’t you infuse data into your existing processes? Make your marketing campaigns run smoother.
Optimize your operations?
Inject it into HR?
I can tell you why you shouldn’t do that: Because you can be confident all of those improvements will be minor improvements. Small improvements won’t pay for the investment, or only very little.
Instead, I suggest you start with opportunities, not problems. Think about all the potential new products, initiatives, and decisions you can develop and make for yourself and your customers.
You can find a good opportunity that provides a significant improvement over the status quo, not just a small incremental one.
Data & AI are different than software products and engineering.
This sentence is used to nudge you to discard common wisdom you’ve gained in a different area, such as product management or team leadership in software engineering.
I don’t hate the general premise, data engineering, ML development, data heavy products, all of those things are of course different from an e-commerce shop.
But so is a banking app.
Or crypto, by a lot.
That’s no good reason to discard common wisdom, especially good business practices, hiring practices, and organizational strategies for success.
My advice is to stick with your experience building great businesses, teams, and products and not discard them prematurely.
Keep them tight until you genuinely see that they don’t work inside your company in your situation.
Be creative about using data.
I hate the “Here’s ten ways you can apply LLMs today in your business” articles with a passion.
They all boil down to the idea of “being creative about using data.”
The idea is that you can embed data, ML, and LLMs into anything, and it will magically improve.
Well, it will not.
Your business isn’t a playground; your career isn’t either. You don’t have to find creative ways to justify an investment.
You want to use generative AI, ML, and all of those tools to advance your core business further, nothing else.
Go For quick wins.
Literally, everyone will tell you to go for a quick win when starting. Be that with business intelligence, data science, or machine learning.
The idea is to “build momentum.”
That’s a pretty metaphor, but it almost always breaks.
Building momentum can be vital if operating with a limited time horizon. But you cannot ignore the more significant goal: To have a big win.
Ideally, you want to have a significant and quick win.
But if you have to choose between a big and a quick win, don’t choose the quick one. Otherwise, your momentum will simply propel you in the wrong direction.
Do any of these ring a bell? If so, please don’t fall for them.
Related Resources
I wrote a couple of pieces on no-bullshit approaches that I think show why these common statements don’t apply to everyone; here they are: