The (Not So Subtle) Art of Not Giving A Fuck About Data
F*ck best practices, data quality, data/ML teams, adversity, BI & Analytics, Data as Product, data & what to do instead.
This is the Three Data Point Thursday, making your business smarter with data & AI.
Want to share anything with me? Hit me up on Twitter @sbalnojan or Linkedin.
In over a decade in data, I've cared deeply about data projects, decision-makers, and machine learning algorithms. Sometimes, I've decided to say fuck it, I didn't give a fuck about best practices, projects, or people. These decisions made all the difference.
I've now spent over a decade in data, cofounded a business intelligence startup (and failed miserably), worked as an analyst, worked inside a hot data startup, led a data team as data PM, and through my writing, have been fortunate to talk to tons of great data founders.
Note: Many of the data founders I've met are reading this; thanks for all the awesome conversations we've had!
One pattern repeated again and again over the past decade:
Companies and managers care about data when they shouldn't. They don't see the forest for the trees. Then nothing comes out of it, so they conclude that data/AI/ML is either too hard to really be of business value or it isn't that important after all.
The one key ability companies and managers need to develop is to control their urge to "do data" They need to not give a fuck about data.
Do you remember the cool kid back in school? The one who seems to not care about anything, not giving a fuck about the girls? And still, he ended up with all of them.
I think you need to be that guy or girl! It's not that he doesn't give a fuck, it's that he has control! It's that he chooses wisely when to give a fuck, so he's able to see the forest.
Because data will suck you right in, into a whirlwind of weird stuff, into giving so many fucks that you're left out dry and ready to die.
These are the fucks you shouldn't give and what to do instead.
No. 1: Giving a fuck about best practices and other companies
Every month or so, Gartner runs a new "hot trend" through the roost. The number of data writers is exploding, and so is the amount of material you get from the AirBnBs, & the Netflixes of this world. All of them love to tell you the best practices of data, of turning a company around, of managing change towards a data-driven culture.
And I do believe what they share is close to their experience.
That doesn't make it a best practice, however. Best practices are the practices others can take and apply successfully to get similar results.
There is no example of another company taking those "best practices" and turning them into business results. Because at the end of the day, that's all that matters, higher growth, or more dollars in the bank. (Not to my knowledge; feel free to correct me!)
Sure, there are tons of companies that "took the best practices from AirBnB" adapted them, and are proud to share what they did. But what none of them share is what they achieved in terms of the company's bottom line.
Because the secret is, it's a big fat negative number in terms of effort and a tiny green number in terms of gain. The online fashion retailer Zalando may have led the data mesh revolution, setting best practices across the market. However, I've yet to find another company that now ships heavy data products faster and thus prints more money because they followed the Zalando best practices. (At least not to our knowledge; feel free to correct!)
The best advice I can give you on how to evaluate hot data trends and what other companies are doing is... to not do it 50% of the time.
No. 2: Giving a fuck about adversity
In the "Subtle Art of Not Giving A Fuck" Mark Manson makes the point that not giving a fuck doesn't mean being indifferent. One meaning is to not care about the adversity and do the right thing.
This is even more true when it comes to working with data. There is precisely one phrase that stands out to me when I think about my decade in data: "This isn't feasible."
Scraping the web for data to sell it to others then? Not feasible for a small start-up (ok, we did fail, but this part we got done...).
Getting analysts into the departments to work with sales? Not feasible.
Building machine learning products and getting them integrated into a legacy frontend? Not feasible.
Turning a data team around to build a platform for others? Not feasible.
Doing product management inside a data team? Not feasible.
I'm not going to lie; data is a lot like oil. BUT not in the sense people think about it, as valuable and powering everything.
Data is like oil because it takes a considerable investment to get it out of the ground. It's a hit-and-miss mission, like drilling oil wells. If you get it out, it's an ugly black matter not ready for consumption, it needs to be refined.
Data is like oil because it's super hard to turn a profit from it - but it is worth it because, in the end, it is super valuable and can power your whole company.
So, what do people do when things are hard? They resist. We're humans, and we all have inertia, a compulsive behavior to resist change. That's normal, and if something, like data, is really hard, the resistance is big.
So to do data right, you'll need to not give a fuck about adversity. But not in the "I'll take an axe to it all" kind of way. You'll need to come in with an understanding but a firm persistence for what's right (data & AI).
You're not going to make friends, but you shouldn't turn others into enemies either.
No. 3: Giving a fuck about data quality
In a company I worked at, I led a project to build an internal tracking system. The idea was to build tracking into all new product features so management could evaluate fast whether they helped to increase the bottom line.
That sounds just like what you'd want to do, right? Except, I spent weeks iterating on the exact format of capturing the data (together with end users), to make it clean, and to make sure the quality is good. So that it could be used with the other data sources we had.
I can tell now when looking back, any tracking would've worked. Hell, we could've used the Google Analytics protocol or even Google Analytics itself. It never was about the quality; the quality turned out to be bad anyhow - because data quality isn't like any other physical product quality.
Data quality = Is the data in a form you need it to be in for the job you have at hand? Thus, data quality isn't like a car; it depends highly on the job, and those differ vastly.
When you give a fuck about data quality, you forget to give a fuck about shipping, about turning data into actions.
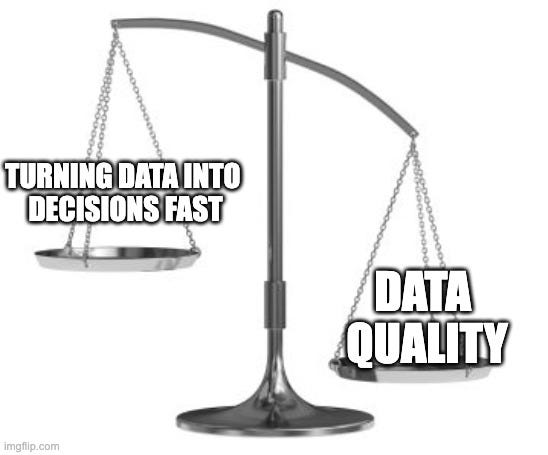
There is only one point in time when you can confidently focus on data quality: It's when you truly think you're a data-driven company. Then you can fine-tune, and increase the little things like quality; before that, ignore it, don't give a fuck.
No. 4: Giving a fuck about BI & analytics
There is a now accepted way of building business intelligence and analytics inside a company.
It starts with hiring a small data team, getting them to collect data into a data warehouse, building out a small modern data stack, and providing dashboards & reports to end users.
On the technical level, it looks like this:
It's the classic fallacy of not seeing the forest for the trees. You don't know how to do data inside your company, but everyone is doing BI, so you hire a data team to get stuff done? And suddenly, you're spending money on tech, and on dashboards, and reports, but not on "BI"”
You should not give a fuck about modern data stacks, or what most people call "BI" and "analytics" Because for some reason, most people do not read the definition:
"Business intelligence (BI) is a set of strategies and technologies enterprises use to analyze business information and transform it into actionable insights that inform strategic and tactical business decisions." (CIO Voice)
The definition is pretty clear and has always been. It's about actions and decisions. Yet, most people only think about the initial part - about getting data and analyzing it, never about the actions or decisions.
If you truly want to do BI and analytics,
you don't start with tech.
You don't start with a data team.
You don't start with analysis.
You start at the end of the pipeline with your marketing & salespeople. With your product managers, with your managers.
You teach them to use data to make decisions; you help them with Excel (yes! Excel), and you help them with the CRM system and the marketing tools you already have. You teach them to use Google Analytics. You use out-of-the-box tools until your decision-makers can confidently work with data to make decisions.
Only then, when the demand for data from all across the company is outgrowing what you can provide out of the box, is the time you should invest in a data team and more tech like a data warehouse.
There is a reason most data teams don't start there: Because it’s hard and messy. But if you don't start with the hard stuff, you're never going to get it done; you're just wasting money.
That's how you do BI & analytics right, by not giving a fuck about how most people think about it. Some people call it "data democratization" but fail to see you don't need to start with a ton of centralized data to then "democratize it" You can teach people to use data immediately in any company!
No. 5: Giving a fuck about data as product
It sometimes looks like people invent ever more points on the "data-product" spectrum. Whenever someone writes, "We got inspired by the data as a product idea," it means they didn't understand the idea.
You can spend your whole career turning datasets into well-curated datasets. You can spend tons of company money on letting data leaders create "data products"
I can tell you, after writing a book about it and reading hundreds of articles on "data products," the truth is pretty simple: There are products, and there is data, period.
To the product manager, the manager, and the business person, there shouldn't be anything in between. It's best to not give a fuck about "data as a product" or "data products" There is a reason people leave the "data" word in front of it because they know what they are talking about is not a true product.
So, what should you care about? Products! Because products do impact your bottom line. Yes, it likely will be better for your bottom line if all your products would be data-heavy, but it doesn't work the other way around! If you just through data into your company at random places, nothing will come out of it but congestion and data-ignorant people.
No. 6: Giving a fuck about small/smart/good data
As we by now should know, big data is dead, and small data and wide data is what most companies now put to use.
Some authors like to introduce good data as the big goal for companies in the mix. Let's return to oil drilling for a second. So here's the thing: oil companies can increase their profit in three ways:
finding new oil fields (the big approach)
among the locations they want to approach, identify the best ones (the small approach)
getting more value out of the oil they are already tapping (the smart approach)
But, the small and smart approach has an obvious ceiling; it all depends on the number of new oil fields.
Once a company becomes smarter in identifying the best oil fields by 20%, it suddenly can invest up to 20% more into finding more oil fields while making the same kind of money.
Once a company becomes 10% better at getting more value out of the oil (by refining better, and integrating oil products into their strategy better), they will invest that profit into finding 10% more oil fields.
No matter where you're at, if you think you're on the smart of the small approach to data, there is only one end game: That's big data. You shouldn't give a fuck about smart, small, wide data. You should never forget about the end game.
That doesn't mean you should only focus on big data, like an oil company; you need a good mix, always switching between getting more inputs vs. optimizing the pipelines you have. But that means you constantly need all three skills and never can forget about the most important one: getting more input.
No. 7: Giving a fuck about data (science/ML) teams
When I stepped up from machine learning engineer to data product manager, it took me months to get rid of my biases. Of my biases for tech and for data, to switch perspectives. And it still keeps pulling me down from time to time.
The reality is this: Data (Science/ML) teams, no matter how you staff them, or how you lead them, will not deliver products. Only product teams will. Data team members and most leaders of data teams will have strong technical biases and less business inclination.
So if you want to build products that are nicely integrated into your company strategy, products that are run on massive amounts of data, you should not give a fuck about data teams.
You should, however care deeply about staffing great product teams with what is needed, and that is:
A business-heavy PM (From my personal experience, I would say a previous engineer is a suboptimal choice here)
a data engineer
a data scientist
a software engineer
UX/frontend engineer
Yes, you might vary the composition, but the point is you need a full product team to build full products, and you need the ability to deal with the data-heavy stuff. You never want to split up the full product team into one building, "just the data", because that team will slip back into the "data as a product" trap.
No. 8: Giving a fuck about data
Do yourself a favor and stop giving a fuck about data. Do give a fuck about the product. If you see a way to make that data-heavy, great, then you should put everything you have behind that and not give a fuck about adversity. If not, don't try to fake it.
The essence is so simple, yet my experience has taught me its also extremely hard.
But then again, all good stuff is, isn't it?
This is such an enlightening and unconventional piece. Thank you for demystifying the whole "data product" thing and for throwing more light on what a true data team should look like and do. And yes small and wide data matters too!