Why Preset.io Is Playing Catch Up And How They Could Reverse The Game
A slight pivot back to the original customer segment yields a completely new roadmap, one that is built on strengths.
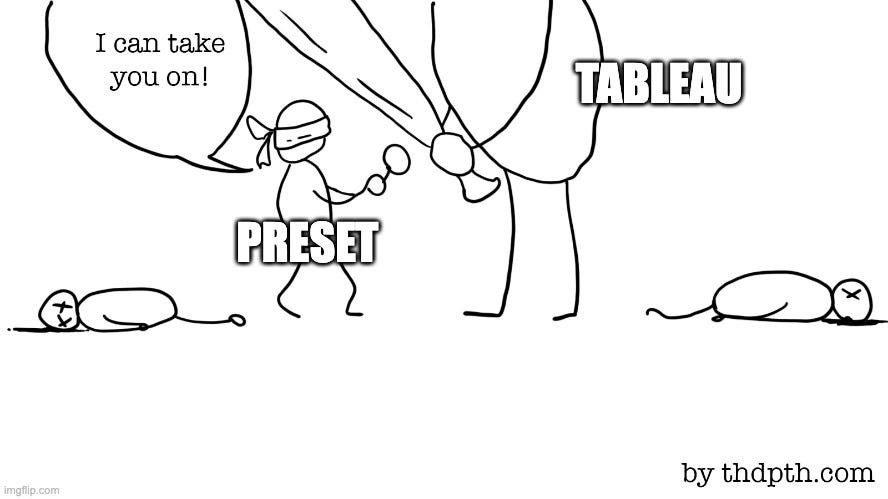
30 billion USD, with a growth rate of about 6% YoY. That’s quite the market size - for the business intelligence software market.
One stellar figure in this space has always been Maxime Beauchemin. A visionary in the data engineering space, who not only worked at Yahoo, Facebook, AirBnB, and more Silicon Valley star companies but also invented Apache Airflow and Apache Superset.
Oh, and he founded and is currently the CEO of the COSS company Preset, which provides a SaaS version of the BI tool Apache Superset.
However, I recently discussed Preset’s product, which left a weird feeling in my stomach. After some research, I realized that I believe Preset is heading in the wrong direction and desperately needs a pivot.
This article discusses that situation.
In Part 1, we will discuss the preset's general situation and the customer problem, the preset's current value proposition, and why it’s about “playing catch-up.” We will then look into the preset’s origins and unique strengths to deduce a better position. We will discuss why we want to fish in a pond where we can be big, not where many big fish are eating us.
In Part 2, we deduce a light, fragmented future roadmap based on a new, different value proposition and go into detail on the new value proposition.
Note: This is an exercise in data product management. It helps me think about the space and might help you think about your own data problems. I do not possess any insight into preset and, as such, can only judge things from the outside, which is hard and likely incorrect or, at the very least, incomplete.
Part 1 - preset basics and a new strategy
Let us understand the origins of preset and deduce a new, better strategy from the unique strengths preset can build on.
A super short history
Preset was founded in 2018 to “democratize business intelligence.” In 2021, the company raised 36m USD in a Series B event. It’s been out of beta since early 2020, providing a hosted superset version with additional features.
The CEO, Maxime, is also the creator of Apache Superset and Apache Airflow. The company was initially very close to the open source project but realized somewhere down the line that it does need to differentiate itself from the free open source project to make money.
It changed the line on its website, “Every feature will always be in Apache superset,” to a more nuanced “preset is a more feature-rich superset” framing.
There’s nothing wrong with that; the mantra for open source should always be
“open up to drive innovation, close down to capture value.”
Now, let us look at the strengths of the company.
Yet, when we compare the usage statistics of Apache Superset and preset, we wonder whether both are doing that well.
If we compare the stackshare references that provide only a sliver of insights, we see a stark picture compared to another open-source project called metabase:
Strengths of preset
But if we look at the star history of the open source project, I think it is safe to say that superset still seems to be one of the favorites.
Superset does have a huge community. It is backed by a genius founder, a genius for advanced data engineering practices. Maxime also pioneered several advanced concepts like Functional Data Engineering, and Dataset-Centric Visualization.
Big companies, including Airbnb and Dropbox, use Superset.
Both Airbnb and Dropbox chose Superset because they have a particular structure for how they work with data: Both companies have strong central expert data engineers who are more than capable of running huge pipelines and providing great data quality to end users. They then have a vast number of decentralized users, analysts, and decision-makers who have a big appetite for data-driven decision-making and thus need this data.
Both companies are large enterprises.
Both companies follow neither a Hub Spoke model nor a Data Mesh concept. This aligns well with Maxime's genius effect as a pioneer of advanced data engineering.
The market & the competition
The BI market is super competitive with a mix of different players. In one segment, we have the legacy players, including Microsoft (Power BI), Google (Looker), Oracle, and SAP.
Another segment is made up of all-in-one solutions like Databricks or AWS.
Open source occupies a significant portion of the market with Superset, Metabase, Redash, and similar tools.
The newcomer lightdash raised 8.4m USD recently.
There’s left-side competition from tools like query books, which offer simple visualization and analysis capabilities. Notebooks, in general, provide another way of solving questions similar to those that BI tools target.
Hex is another player in this field that recently raised another 26m USD, providing a collaborative notebook-like experience.
Finally, the embedded BI tool market has big but hidden players like GoodData.
The technology
Technology dominates the BI market. In particular, I see three current forces that have a lasting impact.
One is AI, and I finally want to get a good AI-assisted query experience. I still haven’t seen one.
Second is the emerging left-field competition. More and more new products emerge that aren’t traditional dashboarding tools but notebooks like Hex canvas tools like count.co or any other new invention.
Third is the underlying technology. BI tools don’t work on their own. They need access to data, which has recently become dominated by dbt. Table formats are becoming a thing, and Databricks and Snowflake keep on fighting for supremacy in the analytics platform market.
The strategy preset needs
We have a fast-moving technology space, a large but scattered and segmented market, and competition in every field.
The dominant strategy to win in such a market is clear: Find a pond where you can be the big fish, a clearly defined and bounded pond. Such boundaries exist because the market is moving so fast. And because the market is so big, there will be ponds with enough customers in it.
The current problem of Preset: For almost two years, Preset has pushed out features that serve the enterprise segment almost exclusively. That’s generally fine but isn’t differentiated. Preset is playing catchup with Tableau and other tools on the market.
Preset is fishing in the big pond of “enterprise customers.” without any boundary applied. But the problem is, in this market, preset will never be a big player, and as such, preset simply cannot win the segment.
Preset is also fishing in a second segment, that of startups with advanced data engineers. Why is that a bad segment? Because it’s cheap! There’s no money to be made with startups. Preset already realized that but hasn’t changed its offering to get away from this segment that still has an influence on the superset project and on Preset’s business.
But you can’t even focus on the big fish when you're busy tendering small fish.
Instead, I’m proposing that Preset pivot to a different customer segment, the original one where Superset still is ahead of the competition: enterprises like Airbnb that have a strong advanced central data engineering team with a data-driven culture and lots of decentralized data users.
This assumption has a lot of implications, and we will discuss it next!
Part 2 - detailed value proposition and a roadmap
We’ve seen why choosing a new customer segment would be beneficial for preset. Or rather returning to the old one they lost over the years.
The goal for the new segment
The enterprises preset should have one clear goal: to enable decentralized data analysis, exploration, and visualization.
The problem for the new segment
The one big problem on their minds is the “time-to-dashboard,” or, if we go a little bit further, getting the “time-to-analysis” down as far as possible.
Thankfully, those companies have a structure that has a clear path to getting the “time-to-analysis” down. Because the analysis happens decentrally inside the business functions, these companies are more than happy to shift load into a central data engineering unit to speed up the “time-to-analysis.”
While many other enterprise features are also on the horizon of these companies, like RBAC, chart labeling and tagging, and collaboration features, they aren’t driving value; they act like constraints. If too many of them are lacking, the enterprise will perceive a drop in value, but one added feature doesn’t increase the value that much.
So, the best strategy is to focus almost exclusively on reducing the “time-to-analysis” while ensuring that you have the bare minimum of enterprise features ready.
Thus, a future roadmap should include at least 60% of “time-to-analysis” features and a maximum of 40% of enterprise-only features.
The issues for the new segment
For our new segment, we can and need to make a few assumptions. We’re assuming we have two parties always involved:
Advanced data engineers, that like best practices, like versioning things, that might even know and be a fan of functional data engineering.
A ton of non-technical analysts and decision makers that don’t even like SQL
We also have an enterprise we have to roll out a solution into and continually add more people. To drive down the “time-to-analysis,” we thus need to cut down the individual times it takes to:
Onboard the company
Onboard new individuals
Get users to understand the data that is connected
Help users find the right questions to ask
Help users answer their question
Help users store and share the answer
Now, let’s finally see what we can do to drive down these numbers, given these strong assumptions!
Potential solutions for the new segment
This is basically a brain dump from me; it’s not meant to be exhausting or even feasible in any way.
But then again, I think all good product work starts with random braindumps.
Diverge farther from open source superset
Preset has already made the difficult decision to develop features that will not be in the open-source version. However, preset should double down on that strategy and push.
All features in this list, all features that strengthen its position into a closed paid preset edition.
And only push the sole features like the 40% enterprise features into the OS superset (or even less)
The reason for this is that, unfortunately, the selected customer segment of advanced data engineers is more than able and eager to self-host their own solution should a managed one not provide a substantial benefit.
I’m considering this feature 0 because it influences all that is about to come.
1. Reverse ETL
If we want to drive down the time to share new numbers an analyst generates, preset could simply add the ability to ship calculated new metrics straight into a CRM or marketing system. That way the analyst would save time sharing data, and others would save time viewing it by using the systems they are already using.
Alternatively, preset could partner up with a hosted reverse ETL solution like Segment.
2. Separating admin and setup tooling
Since we can safely assume a central data engineering team connecting data sources and only analysts creating dashboards, we should remove the admin tooling from the main preset. That way, we declutter the UI and slightly speed up analyst processes.
Increase prices, and make them enterprise-ready!
Let’s discuss pricing. Preset’s current pricing has two tiers, a free tier, and a professional tier, geared towards smaller companies.
That’s because preset doesn’t commit to focusing on enterprises. Instead, a good enterprise pricing tier system could look like this:
Trial (no free tier) phase
3k$/month for medium-sized businesses
user base pricing + setup fee for enterprises
A good pricing structure is like skinny jeans. It fits perfectly for the one target customer segment you have, is perfect for them, and discourages everyone else. Thereby, you can focus 100% of your sales and marketing energy on this one segment and don’t get distracted by customers who want to pay less and clutter your roadmap with features not of use to your high-end customers.
Separate responsibilities, add alerting for new datasets
Analysts shouldn’t need to create new datasets; that should be the job of the central data engineer. Indeed, he should prepare a well-documented dataset—and in our segment, he will!
So, we can take those responsibilities away from analyzing users. Instead, we should add alerting systems that tell all analyzers that a new dataset is connected, including the time of creation.
Preset-specific data format
Now we can get fancy. Since we know our data engineers love best practices and are good with data engineering tooling like dbt, we can ask them to provide all the data for datasets in a specific preset model. That model could include best practices like:
an identifier for the data ingestion source
a timestamp of the last transformation run (hello, functional data engineering!)
more lineage information
more attributes that make working with the data easier for the analyst
We then can expose those attributes in a prominent way inside the dataset explorer and make them searchable!
Integrate way more tools.
Right now, preset integrates nicely with dbt cloud. However, we can safely assume our customer data engineers are fans of tools like Great Expectation or any kind of data catalog.
Enhance dataset browser
The dataset browser is at the heart of the analyst's work. We should provide him with descriptions and quick metrics, such as the count of rows, max-min values, etc., for all data sets. We should also provide him with a sample visualization and point out other graphs other people have built on this dashboard (provide a dashboard and graph lineage).
AI-based explorer
For the sake of it, if you have a complex product like a data explorer, please use AI to help people. Show “what others did” with this data, use gen AI where possible, and provide hints. Don’t rely on people learning your complex feature; make sure even a novice gets the most out of it, without any explanation.
Auto catalog metrics
Right now, preset allows the creation of metrics which are bound to graphs. Metrics are dataset assets and every analyst should see what other metrics are built on top of a dataset. Make metrics a dataset asset and catalog them.
Service!
We’re making setting up preset inside an organization a two-part process, getting data engineers on board, and the decentralized data users.
To make sure all of the preset customers get this process over with as fast as possible, preset should offer a comprehensive onboarding service with clear milestones and consulting to get both sides onto the platform.