🐰 Self-Service at GitLab, Data Mesh at DeliverHero, Data Catalogs vs. Data Discovery; ThDPTh #16 🐰

How self-service analytics works like at GitLab, how DeliverHero built their data mesh with BigQuery, and what you should know about Data Catalogs v.2.0.
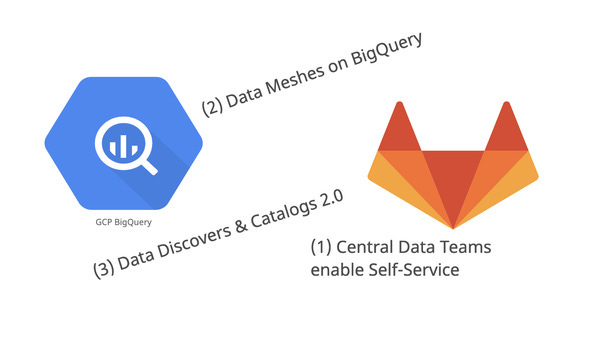
Data will power every piece of our existence in the near future. I collect “Data Points” to help understand & shape this future.
If you want to support this, please share it on Twitter, LinkedIn, or Facebook.
(1) 🔮 GitLabs Data Team & Their Self-Service Program
I just stumbled over the data team at Gitlab. The company Gitlab maintains software I personally enjoy using, they have over 1,000 employees and roughly 130$m in revenue. Of course, I like the openness of GitLab in general, and the precision with which e.g. the data team crafts their team page.
But what I really like is the presentation of their self-service program which I find very fitting for a company of that size. They actively build self-service workflows across the organization, maintain a data catalog and help others conduct their own analysis either dashboard-based with Sisense or plain SQL in Snowflake. Take a look at their program and especially the division of responsibilities they seem to have chosen if you’re at a similar stage of development.
Code, test and deploy with GitLab. Everyone can contribute!
(2)🔥 Presentation by Delivery Hero's Mathias Nitzsche
DeliveryHero, the global food ordering & delivery platform, recently built its own data mesh. And Mathias Nitzsche, a VP of Engineering, shared quite a few insights on the journey as well as the architecture they chose in this presentation with GCP.
What I really like is the architecture using a central BigQuery. Quite a few implementations of the data mesh paradigm actually use a Google BigQuery database but this is one of the first architectures that is explained in detail. The central database is better suited for companies that don’t want to decentralize everything by e.g. using a large AWS S3 based data mesh. And the GCP environment already brings a few additional building blocks that can be used to build the infrastructure layer around BigQuery to support the data mesh.
Customer Spotlight: Delivery Hero
(3)😍 Difference between Data Catalogs and Data Discovery
Barr Moses wrote a good article distinguishing the good old data catalogs, the tools you have to register your data in, from new-age data catalogs which come with data discovery. If you have a data catalog running inside your company you might realize that teams are not really incentivized to keep the catalog up to date if they have to do it by hand for all data sets they produce.
Instead, a new-age approach includes data discovery which focuses on automation and the distributed nature of modern data in microservice architectures & different domains. I recommend reading the article to understand what your current status is and what you really might need, which probably isn’t something teams have to register stuff in by hand.
Data Catalogs Are Dead; Long Live Data Discovery | by Barr Moses | Towards Data Science
For decades, organizations have relied on data catalogs to power data governance. But is that enough?
towardsdatascience.com • Share
🎄 In Other News & Thanks
Thanks for reading this far! I’d also love it if you shared this newsletter with people whom you think might be interested in it.
P.S.: I share things that matter, not the most recent ones. I share books, research papers, and tools. I try to provide a simple way of understanding all these things. I tend to be opinionated. You can always hit the unsubscribe button!
Data; Business Intelligence; Machine Learning, Artificial Intelligence; Everything about what powers our future.
In order to unsubscribe, click here.
If you were forwarded this newsletter and you like it, you can subscribe here.
Powered by Revue