GraphDB Newcomers; Data PM; ML Engineers; ThDPTh #42

I’ve been looking through the database market lately and just stumbled over the fact that I was apparently a bit wrong about terminusDB, and right about the importance of open-source.
Let’s take a look at the whole story…
I’m Sven, I collect “Data Points” to help understand & shape the future, one powered by data, not electricity anymore.
Svens Thoughts
If you only have 30 seconds to spare, here is what I would consider actionable insights for investors, data leaders, and data company founders.
- Five of the six newcomers in the graphDB space are betting on open-source.
- The “data snowflake” problem is a good reason to seriously explore open source as a default option if you’re starting a data company.
- ML engineers are becoming a thing, think seriously about both ways of supporting them and ways of integrating them into your company.
- If you’re doing product management in the data space, internal or external, check out the WSFJ question catalog to fine-tune your value estimations.
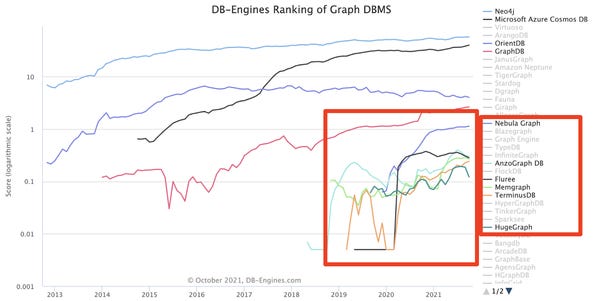
Newcomers in the graph DB space
🔥 What: Since the end of 2018 six new companies entered the graph database space. All of them caught some traction and are breaking into a market that has been dominated by Neo4j for some time, and since 2017 is likely shared between Neo4j and Microsoft’s Cosmos DB.
🐰 My perspective: ⅚ of the newcomers are open-source-based, which I find astonishing. I do believe that in data, open-source should be your default mode of operating, but seeing that is pretty amazing.
What’s also interesting is that the database terminusDB is right up there even though I condemned them (apparently wrongly) as a “dead open source project” at the beginning of this year.
🎁 What: The company Arize.ai discusses the rise of the function of the machine learning engineer. It’s an interesting read about the roles that are still in the shaping in the machine learning/data science space.
🐰 My perspective: I do like the way this article discusses the role of different people inside the ML environment and in particular the ML engineer whose focus it is to make the transition from “data sciency notebook” to “production running system” as smooth as possible.
But I’m not sure the recommendation they follow, to use three specific tools for ML engineering is right. They recommend a feature store, a model store, and an evaluation store.
I’d rather have people harvest the benefits software engineering already brought us and treat data as code, models as code, and simply reuse the tools we already got. From that perspective, the only tools you need are some data versioning solution, all of the good stuff you already got, and some imagination to finally start using what you already do in the scope of machine learning.
Btw. I think these products could have a place, I just think they should be on top of the usual workflow, not trying to replace/ diverge it.
🔮 What: The “weighted shortest job first” method is a not so simple way of prioritizing agile work packages. The idea is to do first what delivers the most value PER unit of time, kind of. If something is valuable and takes a week, something else is valuable and takes two, you do the first one first. It’s that simple.
But that leaves the question of estimating the value. The WSJF method has a catalog of questions I really like to determine that value.
🐰 My perspective: Product management in the data space is in my opinion quite hard. Products are complex, end-users are often businesses, so lots of complexity to start with already. That’s why I like the question catalog, it makes estimating value at least systematic.
The first question is “what is the user-value of this thing?” where I usually add “over the already working alternative?”. So when databricks bought & integrated Redash into the databricks platform, the user-value was not “users are now able to analyze & build dashboards here…”. It was that they didn’t have to go to their external tool and connect it to databricks. Which of course right away shows that the value is actually much smaller because a lot of companies simply enjoy their BI tool.
The second question is “is this urgent?”, which I find particularly important in the reporting & analysis space. Some decision-makers need to make a decision now because of some deadline, which simply makes the task really important. But even if it is really important, every decision-maker can also make his decision without an analysis. So again, time criticality is relative.
Finally, we ask for risk reduction & opportunity enablement; Adding an option to host data inside the EU for instance reduces the risk of European companies getting sued for breaking the GDPR.
I think data product management still needs more rigor, and I think this is one good step in that direction.
www.scaledagileframework.com • Share
🎄 Thanks!
Thanks for reading this far! I’d also love it if you shared this newsletter with people whom you think might be interested in it.
Data will power every piece of our existence in the near future. I collect “Data Points” to help understand & shape this future.
If you want to support this, please share it on Twitter, LinkedIn, or Facebook.
And of course, leave feedback if you have a strong opinion about the newsletter! So?
It is terrible | It’s pretty bad | average newsletter… | good content… | I love it, will forward!!!
P.S.: I share things that matter, not the most recent ones. I share books, research papers, and tools. I try to provide a simple way of understanding all these things. I tend to be opinionated. You can always hit the unsubscribe button!
Data; Business Intelligence; Machine Learning, Artificial Intelligence; Everything about what powers our future.
In order to unsubscribe, click here.
If you were forwarded this newsletter and you like it, you can subscribe here.
Powered by Revue